Interview with Marion Cabrol
Data & AI Stories: what is a Data Scientist?
Data and artificial intelligence are cornerstones of our digitalisation strategy and support our three strategic priorities: client centricity, operational efficiency and responsibility. In 2019, we entered a successful scaling phase as evidenced by our portfolio of 250 data & AI use cases in production. The current challenge for Societe Generale is to accelerate this scaling strategy. In order to do this, the Group relies on multidisciplinary teams with the ability to quickly develop effective and reusable data and AI solutions. We would like to invite you to take a look.
Today's spotlight is on Marion Cabrol, Data scientist at the Coverage and Investment Banking Digital Office.
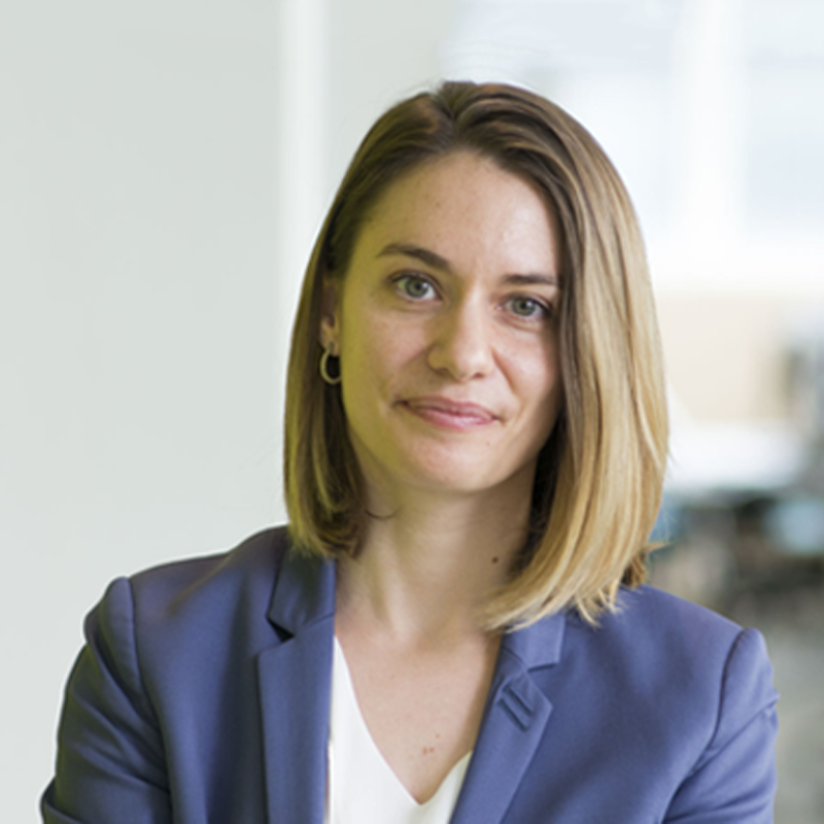
How would you explain your job "for dummies"?
I gather data, and using maths and IT tools, I ‘guess' information. You know how Amazon and Netflix guess which books, films or TV series interest you? Well, behind that guess is a data scientist who looks at your recent purchase data, Netflix favourites and history! A data scientist in the financial sector, for example, could guess which financial products would suit their client best.
Which other business, IT and data divisions do you collaborate with in the Group?
I work a lot with people in business because they are more in a position to contextualise the problem that needs solving with data science. I am in contact with the business lines all throughout the project's development: whether it's to better understand the data provided or to test data-science models, for example, we need their feedback. Our team also includes data engineers which enable us to put our machine-learning models into production (preparing data, APIs that are suited to machine learning, developing more user-friendly graphic interfaces, etc.).
What are some examples of the use cases you work on?
I worked on a budget assistant to improve operational efficiency. It helps employees prepare the Global Banking and Advisory budget by making predictions for certain portions of the budget according to various economic scenarios. We are also working on a project to automatically detect information in fund prospectuses at Securities Services (Societe Generale Securities Services). This information is vital for the business line, which is accustomed to manually retrieving roughly 50 details from prospectuses ranging from several dozen to several hundred pages.
The term artificial intelligence (AI) is everywhere these days. Is it anything new?
This field is by no means new. Scientists had begun working on AI by the 1950s. But over the past few years, two phenomena have helped expand the possibilities of artificial intelligence and refine its results – the data boom and technical innovations for increasingly sophisticated AI algorithms.
Many companies, financial or other, use AI as a pillar of their strategy because it enables them to automate repetitive tasks and develop new business lines. In finance, we are also lucky to have a plethora of data in very different formats, so AI offers a great deal of opportunities.
In your opinion, where is Societe Generale at with its scaling efforts?
Considering that this company started 156 years ago and encompasses 138,000 employees in 62 countries, with many information systems and clients across numerous countries in different jurisdictions, scaling is no small feat. How can we be sure that the project developed in Paris is suitable for every jurisdiction in every country? How can we be sure someone else hasn't developed a similar solution on the other side of world?
The Data Science teams actively take part in amplifying the scale up efforts that are already in progress at Societe Generale. Our teams are centralised with the right skills and expertise, and collaborate closely with the business lines so that we can quickly accommodate business-line use cases. Within the data-science community, we regularly share the field's latest achievements and communicate a lot about reusing solutions that have been tried and tested. The majority of code from projects is made available to the various data labs. Most of the bank's AI projects are centralised and accessible on our dedicated internal platform.
See Marion Cabrol during the digital event AI For Finance, on Thursday, 10th September. She will participate in the meet-up Women in Machine Learning and Data Science (WiMLDS) on the subject "Transforming data into value in finance."